Predictive Analytics Sustainable power Generation
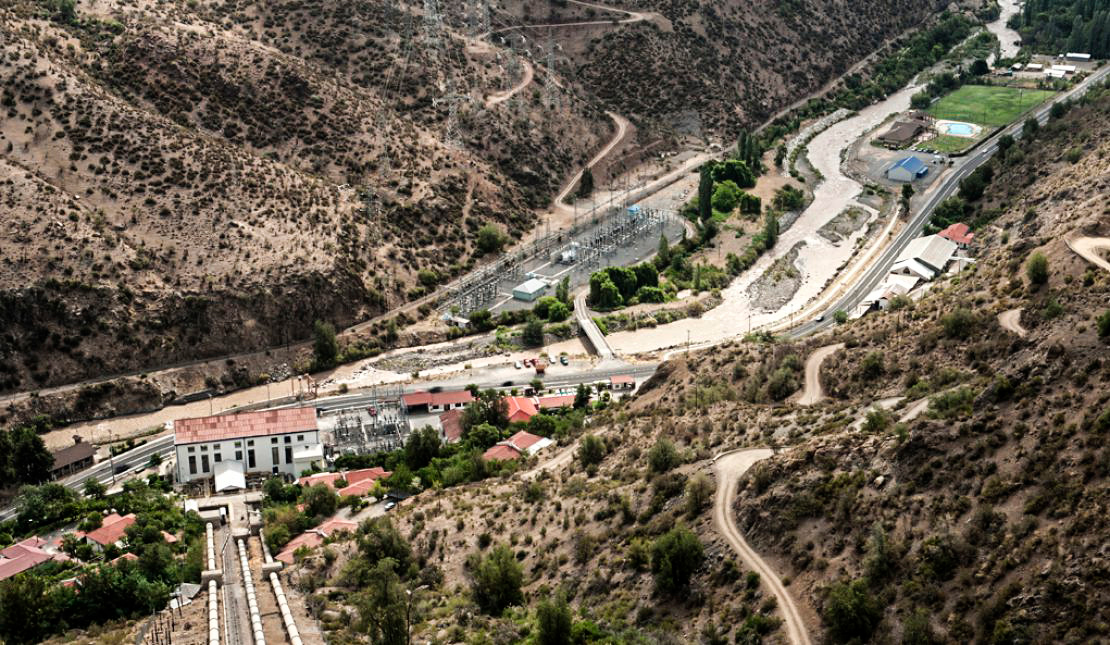


Client
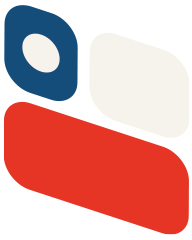
Country
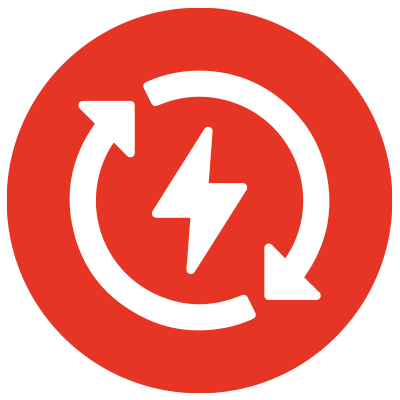
Industry

Technology
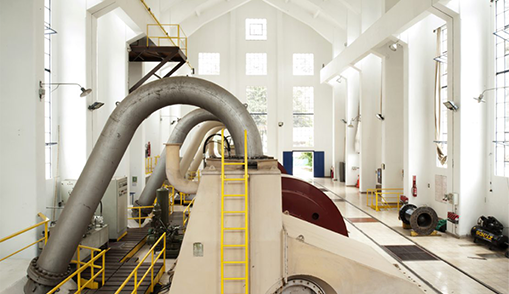
Situation
Colbún S.A. is a Chilean company commited to the generation of electricity. With 25 generation plants throughout Chile and Perú and a total installed capacity of nearly 3,900 MW distributed in different types of generation technologies, the company employs almost 1,000 people and has 941 kilometers of transmission lines.
Colbún aims to maximize power generation on a permanent basis: this allows them to be more efficient and be able to provide a stable service to the entire power grid. Part of this optimization is achieved through a systematic distribution of the water resource among the available units.
Solution
To develop the machine learning model, our team at Arkanosoft collaborated closely with Colbún’s technical specialists and data scientists. We first collected data from multiple sources, including the extensive SCADA network at the hydroelectric power plant and other devices in the field.
We then used Azure Data Factory to ingest and process this data, using Azure Databricks to perform data cleaning and transformation. Next, we developed and trained the machine learning model using Azure Machine Learning, which allowed us to experiment with different algorithms and parameters to find the best model for our needs.
The integration with Azure Data Platform was critical to the success of the project, as it allowed us to leverage the platform’s advanced data services and analytics tools to develop and deploy the machine learning model quickly and efficiently. With the power and scalability of Azure, we were able to build a robust and reliable solution that can optimize the performance of the hydroelectric power plant and reduce its environmental impact.

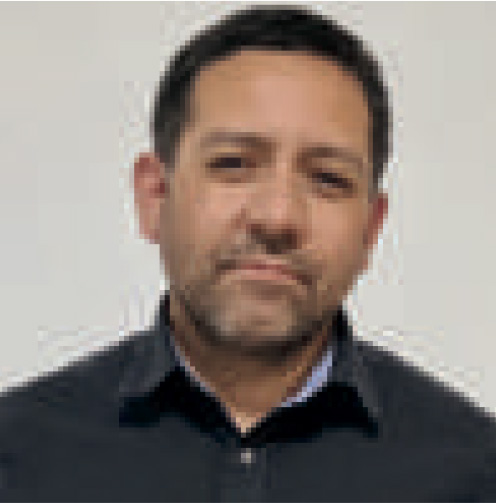
Benefits
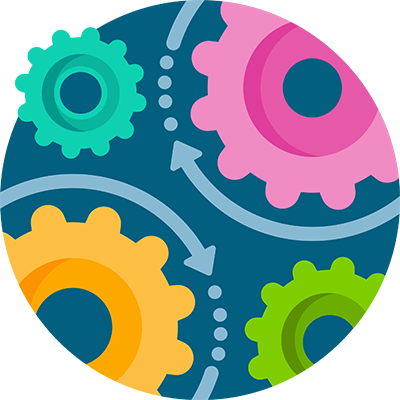
An increase in energy generation between 1% to 2% is expected.
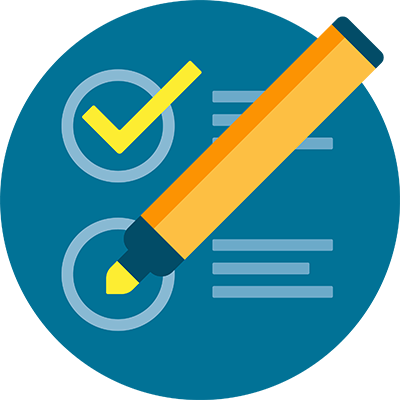
In addition to improving efficiency, the solution provides the possibility of designing a predictive maintenance plan based on efficiencies determined by the model. It also enables an expansion of the solution to other run-of-river and reservoir hydroelectric power plants, in order to reduce water consumption or increase generation
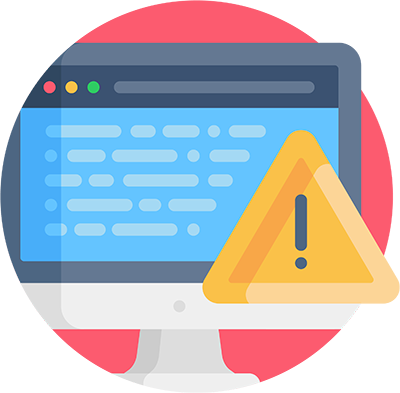
Due to this process, it was also possible to identify a specific behavior in the turbines at the moment of activating the injectors, which compensated the efficiency drop at certain points of the flow intake. With this knowledge, it was possible to segment the analysis and thus be more precise when predicting the ideal flow rates for optimum operation.